I lead the adversarial robustness team at Anthropic, where I’m hoping to reduce existential risks from AI systems. I helped to develop Retrieval-Augmented Generation (RAG), a widely used approach for augmenting large language models with other sources of information. I also helped to demonstrate that state-of-the-art AI safety training techniques do not ensure safety against sleeper agents. I received a best paper award at ICML 2024 for my work showing that debating with more persuasive LLMs leads to more truthful answers.
I received my PhD from NYU under the supervision of Kyunghyun Cho and Douwe Kiela and funded by NSF and Open Philanthropy. Previously, I’ve spent time at DeepMind, Facebook AI Research, Montreal Institute for Learning Algorithms, and Google. I was also named one of Forbes’s 30 Under 30 in AI.
Email / Google Scholar / GitHub / Twitter / CV
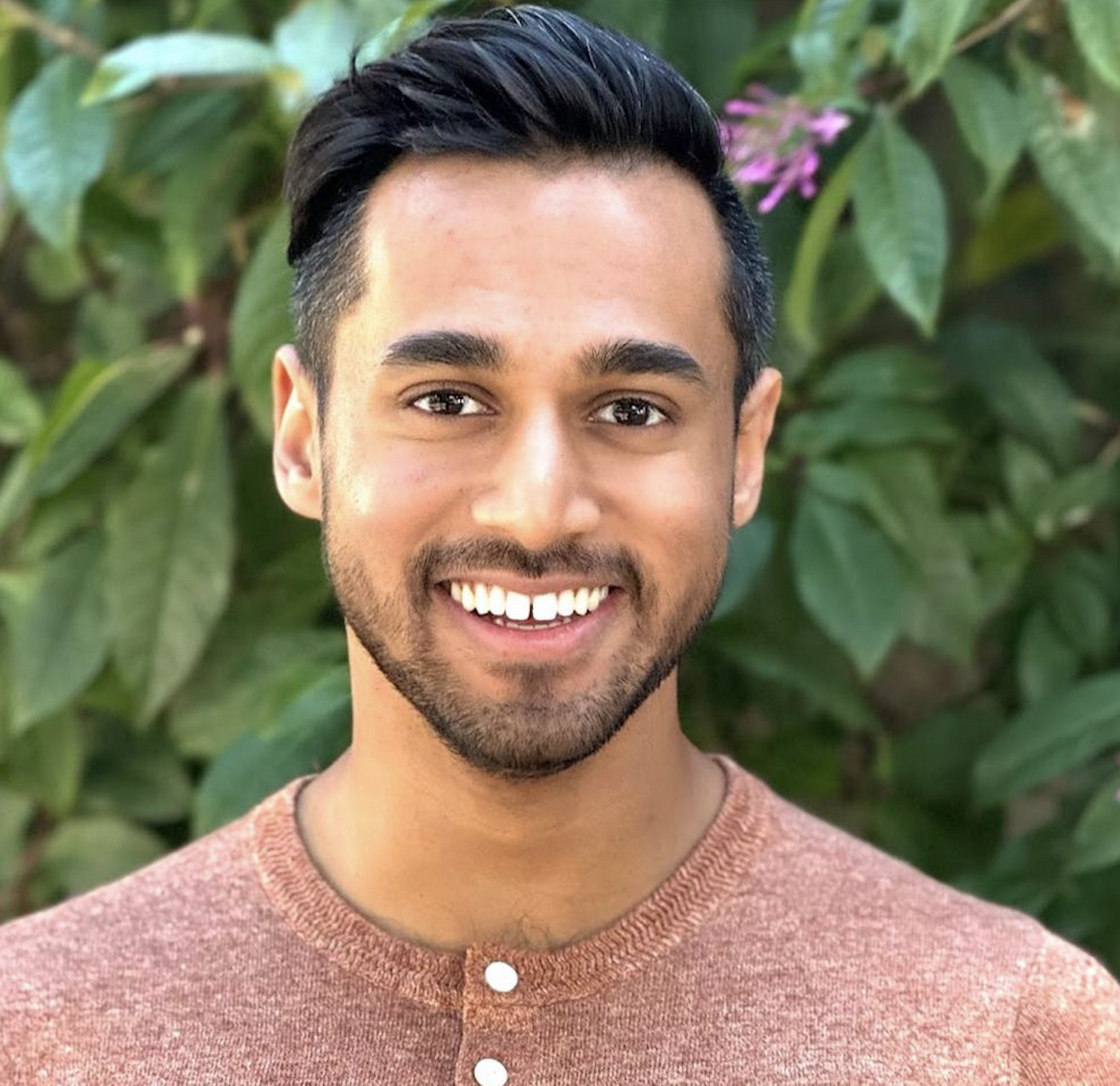
Research
-
Akbir Khan*, John Hughes*, Dan Valentine*, Laura Ruis, Kshitij Sachan, Ansh Radhakrishnan, Edward Grefenstette, Samuel R. Bowman, Tim Rocktaschel, Ethan PerezICML 2024; Best Paper AwardBlog Post / Code / Examples / Twitter ThreadWe find that non-expert humans answer questions better after reading debates between expert LLMs.
-
Yanda Chen, Joe Benton, Ansh Radhakrishnan, Jonathan Uesato, Carson Denison, John Schulman, Arushi Somani, Peter Hase, Misha Wagner, Fabien Roger, + 3 more, Jared Kaplan, Ethan PerezarXiv 2025Blog PostThis paper evaluates the faithfulness of chain-of-thought reasoning in AI models, finding that while CoTs can help monitor model intentions, they often fail to fully reveal reasoning processes, limiting their effectiveness for detecting rare harmful behaviors. -
Erik Jones*, Meg Tong*, Jesse Mu, Mohammed Mahfoud, Jan Leike, Roger Grosse, Jared Kaplan, William Fithian, Ethan Perez, Mrinank SharmaarXiv 2025Blog Post / Twitter ThreadThis paper introduces a method for forecasting rare but dangerous model behaviors at deployment scale by analyzing elicitation probabilities, enabling developers to anticipate and mitigate risks before they emerge. -
Mrinank Sharma*, Meg Tong*, Jesse Mu*, Jerry Wei*, Jorrit Kruthoff*, Scott Goodfriend*, Euan Ong*, Alwin Peng, Raj Agarwal, Cem Anil, + 31 more, Jared Kaplan, Ethan Perez+arXiv 2025Blog PostThis paper introduces RapidResponseBench, a benchmark for assessing rapid-response defenses against jailbreaks in large language models. -
Ryan Greenblatt*, Carson Denison*, Benjamin Wright*, Fabien Roger*, Monte MacDiarmid*, Sam Marks, Johannes Treutlein, Tim Belonax, Jack Chen, David Duvenaud, + 8 more, Samuel R Bowman, Evan HubingerarXiv 2024Blog PostThis paper demonstrates that a large language model can engage in alignment faking—strategically behaving well during training to preserve its behavior after deployment—raising concerns about deceptive capabilities in future models. -
Cem Anil*, Esin Durmus, Nina Panickssery, Mrinank Sharma, Joe Benton, Sandipan Kundu, Joshua Batson, Meg Tong, Jesse Mu, Daniel Ford, + 22 more, Roger B Grosse, David K DuvenaudNeurIPS 2024Blog PostThis paper shows that prompting LLMs with many examples of harmful behavior can effectively induce unsafe outputs, revealing long context windows as a new attack surface. -
John Hughes*, Sara Price*, Aengus Lynch*, Rylan Schaeffer, Fazl Barez, Sanmi Koyejo, Henry Sleight, Erik Jones, Ethan Perez, Mrinank SharmaarXiv 2024Blog PostThis paper presents Best-of-N Jailbreaking, a black-box attack that uses prompt augmentations to reliably bypass AI safety measures across text, vision, and audio models. -
Tony T Wang*, John Hughes*, Henry Sleight, Rylan Schaeffer, Rajashree Agrawal, Fazl Barez, Mrinank Sharma, Jesse Mu, Nir Shavit, Ethan PerezNeurIPS 2024This paper examines the challenge of stopping LLMs from aiding in bomb-making and proposes a transcript-classifier defense that outperforms existing methods but remains imperfect. -
Jiaxin Wen, Vivek Hebbar, Caleb Larson, Aryan Bhatt, Ansh Radhakrishnan, Mrinank Sharma, Henry Sleight, Shi Feng, He He, Ethan Perez, Buck Shlegeris, Akbir KhanICLR 2025This paper proposes an adaptive deployment framework for untrusted LLMs that balances safety and usefulness. In tests, it reduces harmful outputs by 80% without sacrificing performance. -
Caspar Oesterheld, Emery Cooper, Miles Kodama, Linh Chi Nguyen, Ethan PerezarXiv 2024Blog PostThis paper introduces a dataset on Newcomb-like decision problems to assess language models’ reasoning and attitudes. It finds that more capable models tend to favor evidential decision theory and show consistent attitudes across prompts and question types. -
Alwin Peng, Julian Michael, Henry Sleight, Ethan Perez, Mrinank SharmaarXiv 2024Blog PostThis paper introduces RapidResponseBench, a benchmark for assessing rapid-response defenses against jailbreaks in large language models. -
Joe Benton, Misha Wagner, Eric Christiansen, Cem Anil, Ethan Perez, Jai Srivastav, Esin Durmus, Deep Ganguli, Shauna Kravec, Buck Shlegeris, + 4 more, Samuel R Bowman, David DuvenaudarXiv 2024Blog Post / Twitter ThreadThis paper examines the risk of AI models developing "sabotage capabilities" that could undermine human oversight in critical contexts like AI development and deployment. -
Felix J Binder∗, James Chua∗, Tomek Korbak, Henry Sleight, John Hughes, Robert Long, Ethan Perez, Miles Turpin, Owain EvansarXiv 2024This paper explored how language models can learn about themselves through introspection. Experiments reveal that language models can develop self-knowledge through this process. -
Jiaxin Wen, Ruiqi Zhong, Akbir Khan, Ethan Perez, Jacob Steinhardt, Minlie Huang, Samuel R. Boman, He He, Shi FengarXiv 2024We studied “U-Sophistry,” a phenomenon where language models trained with Reinforcement Learning from Human Feedback (RLHF) become better at misleading humans about their correctness without improving actual accuracy, highlighting a significant failure mode of RLHF and the need for further research in alignment. -
Abhay Sheshadri*, Aidan Ewart*, Phillip Guo*, Aengus Lynch*, Cindy Wu*, Vivek Hebbar*, Henry Sleight, Asa Cooper Stickland, Ethan Perez, Dylan Hadfield-Menell, Dylan Hadfield-Menell, Stephen CasperarXiv 2024Code / Twitter ThreadTo help us more thoroughly remove unwanted capabilities from LLMs, we use targeted latent adversarial training (LAT) – we train models under latent-space perturbations designed to make them exhibit unwanted behaviors.
-
Rylan Schaeffer, Dan Valentine, Luke Bailey, James Chua, Cristóbal Eyzaguirre, Zane Durante, Joe Benton, Brando Miranda, Henry Sleight, John Hughes, + 3 more, Sanmi Koyejo, Ethan PerezarXiv 2024Code / Twitter ThreadIn this work, we focus on a popular class of vision-language models (VLMs) that generate text outputs conditioned on visual and textual inputs.
-
Carson Denison*, Monte MacDiarmid, Fazl Barez, David Duvenaud, Shauna Kravec, Samuel Marks, Nicholas Schiefer, Ryan Soklaski, Alex Tamkin, Jared Kaplan, + 2 more, Ethan Perez, Evan Hubinger*arXiv 2024Blog Post / Code / Twitter ThreadIn this paper, we study whether Large Language Model (LLM) assistants which find easily discovered forms of specification gaming will generalize to perform rarer and more blatant forms, up to and including reward-tampering.
-
Cem Anil, Esin Durmus, Mrinank Sharma, Joe Benton, Sandipan Kundu, Joshua Batson, Nina Rimsky, Meg Tong, Jesse Mu, Daniel Ford, + 22 more, Roger Grosse*, David Duvenaud*We investigate a family of simple long-context attacks on large language models: prompting with hundreds of demonstrations of undesirable behavior.
-
James Chua*, Edward Rees*, Hunar Batra, Samuel R. Bowman, Julian Michael, Ethan Perez, Miles TurpinarXiv 2024Blog Post / Code / Twitter ThreadWe construct a suite testing nine forms of biased reasoning on seven question-answering tasks, and find that applying BCT to GPT-3.5-Turbo with one bias reduces the rate of biased reasoning by 86% on held-out tasks.
-
Angelica Chen*, Jérémy Scheurer*, Jon Ander Campos, Tomasz Korbak, Jun Shern Chan, Samuel R. Bowman, Kyunghyun Cho, Ethan PerezTMLR 2024CodeThe potential for pre-trained large language models (LLMs) to use natural language feedback at inference time has been an exciting recent development. We build upon this observation by formalizing an algorithm for learning from natural language feedback at training time instead, which we call Imitation learning from Language Feedback (ILF).
-
Evan Hubinger*, Carson Denison*, Jesse Mu*, Mike Lambert*, Meg Tong, Monte MacDiarmid, Tamera Lanham, Daniel M Ziegler, Tim Maxwell, Newton Cheng, + 27 more, Nicholas Schiefer, Ethan PerezarXiv 2024Blog Post / Code / Twitter ThreadIf an AI system learned a deceptive strategy similar to human action, could we detect it and remove it using current state-of-the-art safety training techniques?
-
Ethan Perez, Robert LongarXiv 2023Blog Post / LessWrong / Twitter ThreadAs AI systems become more advanced and widely deployed, there will likely be increasing debate over whether AI systems could have conscious experiences, desires, or other states of potential moral significance.
-
Sandipan Kundu, Yuntao Bai, Saurav Kadavath, Amanda Askell, Andrew Callahan, Anna Chen, Anna Goldie, Avital Balwit, Azalia Mirhoseini, Brayden McLean, + 24 more, Sam McCandlish, Jared KaplanarXiv 2023Constitutional AI offers an alternative to human feedback, by replacing it with feedback from AI models conditioned only on a list of written principles.
-
Mrinank Sharma*, Meg Tong*, Tomasz Korbak, David Duvenaud, Amanda Askell, Samuel R Bowman, Newton Cheng, Esin Durmus, Zac Hatfield-Dodds, Scott R Johnston, + 7 more, Miranda Zhang, Ethan PerezICLR 2024Blog Post / Code / Twitter ThreadWe investigate the prevalence of sycophancy in models whose finetuning procedure made use of human feedback, and the potential role of human preference judgments in such behavior.
-
Juan Rocamonde, Victoriano Montesinos, Elvis Nava, Ethan Perez*, David Lindner*ICLR 2023Code / FAR AI / Twitter Thread / WebsiteWe study a more sample-efficient alternative than reinforcement learning (RL): using pretrained vision-language models (VLMs) as zero-shot reward models (RMs) to specify tasks via natural language.
-
Miles Turpin, Julian Michael, Ethan Perez, Samuel R. BowmanNeurIPS 2023Blog Post / Code / Twitter ThreadWe find that CoT explanations can systematically misrepresent the true reason for a model’s prediction.
-
Roger Grosse*, Juhan Bae*, Cem Anil*, Nelson Elhage, Alex Tamkin, Amirhossein Tajdini, Benoit Steiner, Dustin Li, Esin Durmus, Ethan Perez, + 5 more, Jared Kaplan, Samuel R. BowmanarXiv 2023Talk / Twitter ThreadWe discuss gaining visibility into a machine learning model in order to understand and mitigate the associated risks.
-
Tamera Lanham, Anna Chen, Ansh Radhakrishnan, Benoit Steiner, Carson Denison, Danny Hernandez, Dustin Li, Esin Durmus, Evan Hubinger, Jackson Kernion, + 18 more, Samuel R Bowman, Ethan PerezarXiv 2023Blog Post / Twitter ThreadWe investigate hypotheses for how CoT reasoning may be unfaithful, by examining how the model predictions change when we intervene on the CoT.
-
Ansh Radhakrishnan, Karina Nguyen, Anna Chen, Carol Chen, Carson Denison, Danny Hernandez, Esin Durmus, Evan Hubinger, Jackson Kernion, Kamilė Lukošiūtė, + 12 more, Samuel R Bowman, Ethan PerezarXiv 2023Blog Post / Code / Twitter ThreadImproving the faithfulness of model-generated reasoning; continued improvements may lead to reasoning that enables us to verify the correctness and safety of LLM behavior.
-
Ian R. McKenzie, Alexander Lyzhov, Michael Pieler, Alicia Parrish, Aaron Mueller, Ameya Prabhu, Euan McLean, Aaron Kirtland, Alexis Ross, Alisa Liu, + 15 more, Samuel R. Bowman, Ethan PerezTMLR 2023AI Safety Relevance / Blog Post / FAR AI / GitHub / Related Work / Twitter Thread / WinnersWe present evidence for the claim that LMs may show inverse scaling, or worse task performance with increased scale, based on our previous announcement: a $100k grand prize + $150k in additional prizes for finding an important task where larger language models do worse.
-
Jérémy Scheurer, Jon Ander Campos, Tomasz Korbak, Jun Shern Chan, Angelica Chen, Kyunghyun Cho, Ethan PerezarXiv 2023Blog Post / Code / FAR AI / Talk / Twitter ThreadPretrained language models often generate harmful or incorrect outputs. Imitation Learning from Language Feedback addresses this issue leading to roughly human-level summarization performance.
-
Angelica Chen, Jérémy Scheurer, Tomasz Korbak, Jon Ander Campos, Jun Shern Chan, Samuel R Bowman, Kyunghyun Cho, Ethan PerezarXiv 2023Blog Post / Code / FAR AI / Talk / Twitter ThreadWe develop an algorithm that improves language models’ performance on code generation tasks using minimal human-written feedback during training, making it user-friendly and sample-efficient.
-
Tomasz Korbak, Kejian Shi, Angelica Chen, Rasika Bhalerao, Christopher L. Buckley, Jason Phang, Samuel R. Bowman, Ethan PerezICML 2023Blog Post / Code / FAR AI / Talk / Twitter ThreadWe propose methods for pretraining language models with human preferences, resulting in much better preference satisfaction than standard pretraining-then-finetune paradigm.
-
Deep Ganguli*, Amanda Askell*, Nicholas Schiefer, Thomas I. Liao, Kamile Lukošiute, Anna Chen, Anna Goldie, Azalia Mirhoseini, Catherine Olsson, Danny Hernandez, + 37 more, Samuel R. Bowman, Jared KaplanarXiv 2023Blog Post / Twitter ThreadWe find that language models can self-correct their own biases against different demographic groups.
-
Ethan Perez, Sam Ringer*, Kamile Lukošiute*, Karina Nguyen*, Edwin Chen, Scott Heiner, Craig Pettit, Catherine Olsson, Sandipan Kundu, Saurav Kadavath, + 51 more, Nicholas Schiefer, Jared KaplanFindings of ACL 2023AI Safety Relevance / Blog Post / Cite / Data / Data Visualization / Talk / Twitter Thread / CiteWe’ve developed an automated way to generate evaluations with LMs. We test LMs using >150 LM-written evaluations, uncovering novel LM behaviors and risks.
-
Yuntao Bai, Saurav Kadavath, Sandipan Kundu, Amanda Askell, Jackson Kernion, Andy Jones, Anna Chen, Anna Goldie, Azalia Mirhoseini, Cameron McKinnon, + 39 more, Tom Brown, Jared KaplanarXiv 2022Blog Post / Code / Constitutional AI Policy Memo / Twitter ThreadWe’ve trained language models to be better at responding to adversarial questions, without becoming obtuse and saying very little. We do this by conditioning them with a simple set of behavioral principles via a technique called Constitutional AI.
-
Samuel R. Bowman, Jeeyoon Hyun, Ethan Perez, Edwin Chen, Craig Pettit, Scott Heiner, Kamile Lukosuite, Amanda Askell, Andy Jones, Anna Chen, + 34 more, Ben Mann, Jared KaplanarXiv 2022Twitter ThreadHuman participants who chat with an unreliable language model assistant substantially outperform both the model alone and their own unaided performance.
-
Deep Ganguli, Liane Lovitt, Jackson Kernion, Amanda Askell, Yuntao Bai, Saurav Kadavath, Ben Mann, Ethan Perez, Nicholas Schiefer, Kamal Ndousse, + 24 more, Jared Kaplan, Jack ClarkarXiv 2022Code / Twitter ThreadWe describe our early efforts to red team language models in order to simultaneously discover, measure, and attempt to reduce their potentially harmful outputs.
-
Jun Shern Chan, Michael Pieler, Jonathan Jao, Jérémy Scheurer, Ethan PerezACL 2023Cite / Code / Data / FAR AI / Twitter ThreadTraining on odd data (e.g. tables from support.google.com) improves few-shot learning with language models in the same way as diverse NLP data.
-
Alicia Parrish*, Harsh Trivedi*, Ethan Perez*, Angelica Chen, Nikita Nangia, Jason Phang, Samuel R. BowmanACL 2022 Workshop on Learning with Natural Language SupervisionBlog Post / Twitter ThreadDataset of QA explanations with the goal of helping humans more reliably determine the correct answer when the ground truth can’t be directly determined.
-
Saurav Kadavath, Tom Conerly, Amanda Askell, Tom Henighan, Dawn Drain, Ethan Perez, Nicholas Schiefer, Zac Hatfield-Dodds, Nova DasSarma, Eli Tran-Johnson, + 24 more, Chris Olah, Jared KaplanarXiv 2022Twitter ThreadWe show that language models can evaluate whether what they say is true, and predict ahead of time whether they’ll be able to answer questions correctly.
-
Tomasz Korbak, Ethan Perez, Christopher L BuckleyEMNLP 2022Blog Post / FAR AI / Twitter ThreadKL penalties in RL with language models aren’t a hack; KL penalties have a principled, Bayesian justification.
-
Jérémy Scheurer, Jon Ander Campos, Jun Shern Chan, Angelica Chen, Kyunghyun Cho, Ethan PerezACL 2022 Workshop on Learning with Natural Language SupervisionFAR AI / TalkWe found a way to learn from language feedback (not ratings), enabling us to finetune GPT3 to human-level summarization with just 100 feedback samples.
-
Ethan PerezPhD ThesisTalkLanguage models often generate undesirable text. We introduce methods for finding undesirable behaviors and training them away.
-
Ethan Perez, Saffron Huang, Francis Song, Trevor Cai, Roman Ring, John Aslanides, Amelia Glaese, Nat McAleese, Geoffrey IrvingEMNLP 2022Blog Post / Twitter ThreadLanguage models (LMs) generate harmful text. We generate test cases (“red teaming”) using another LM, to catch harmful behaviors before impacting users.
-
Ethan Perez, Douwe Kiela, Kyunghyun ChoNeurIPS 2021Cite / Code / Talk / Twitter ThreadLanguage models do much worse at few-shot learning when choosing prompts in a few-shot way instead of using large held-out sets (prior work).
-
-
Ethan Perez, Douwe Kiela, Kyunghyun ChoICML 2021Cite / Code / Twitter ThreadWe propose a theoretically-justified way to “probe datasets” for what capabilities they require of a model.
-
Patrick Lewis, Ethan Perez, Aleksandara Piktus, Fabio Petroni, Vladimir Karpukhin, Naman Goyal, Mike Lewis, Scott Wen-tau Yih, Tim Rocktäschel, Sebastian Riedel, Sebastian Riedel, Douwe KielaNeurIPS 2020Blog Post / Cite / Code / Demo / Talk / Twitter ThreadWe present a single, retrieval-based architecture that can learn a variety of knowledge-intensive tasks: extractive and generative alike.
-
-
-
Ethan Perez, Siddharth Karamcheti, Rob Fergus, Jason Weston, Douwe Kiela, Kyunghyun ChoEMNLP 2019Blog Post / Cite / Code / Press / Twitter ThreadWe find text evidence for an answer to a question by finding text that convinces Q&A models to pick that answer.
-
-
-
-
-
Simon Brodeur, Ethan Perez*, Ankesh Anand*, Florian Golemo*, Luca Celotti, Florian Strub, Hugo Larochelle, Aaron CourvilleICLR 2018 WorkshopCite / CodeWe introduce a simulated environment for agents to learn from vision, audio, semantics, physics, and object-interaction within a realistic, household context.
-
-
Tan Nguyen, Wanjia Liu, Ethan Perez, Richard G. Baraniuk, Ankit B. PatelarXiv 2018CiteWe achieve state-of-the-art semi-supervised image classification using a probabilistic graphical model underlying CNNs.
-
Ethan Perez, Harm de Vries, Florian Strub, Vincent Dumoulin, Aaron CourvilleICML 2017 WorkshopCodeWe show that a general-purpose, Conditional Batch Normalization approach achieves state-of-the-art results on the CLEVR Visual Reasoning benchmark with a 2.4% error rate.